- Forbes
- December 3, 2024
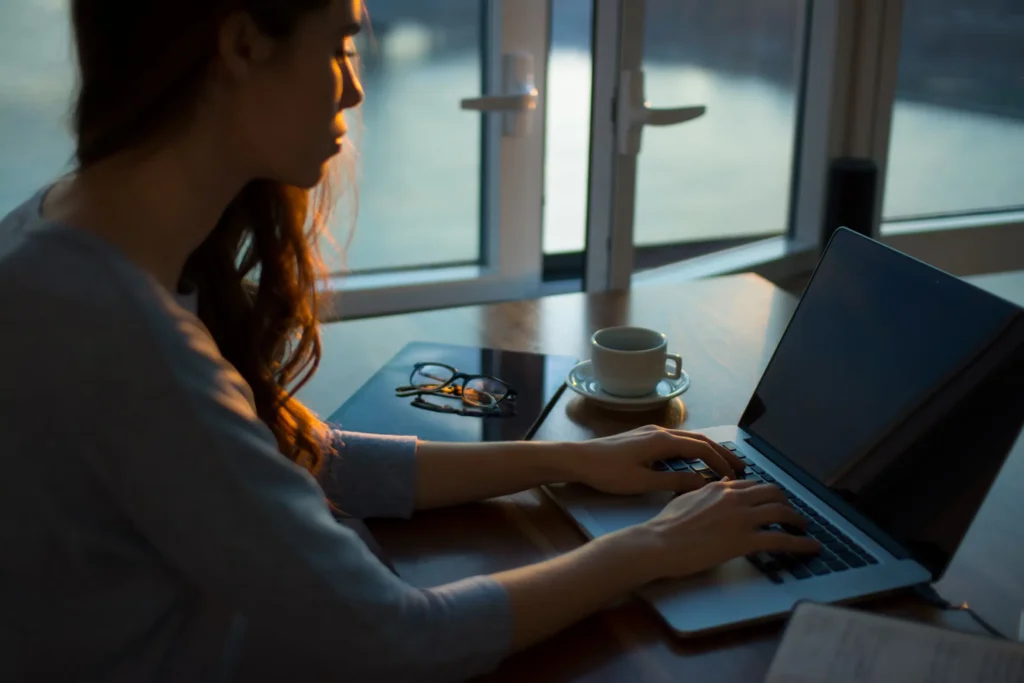
Dan Galorath is the founder and CEO of Galorath, a leader in cost estimation, actionable data and expertise for informed decision-making.
Artificial intelligence (AI) is transforming the business landscape, revolutionizing workflows, and changing how we analyze data and access information. According to a recent survey, the AI market is projected to reach a staggering $407 billion by 2027, experiencing substantial growth from its estimated $87 billion revenue in 2022. In addition, a recent Forbes Advisor survey notes that 64% of businesses believe AI will help increase overall productivity.
Yet when leveraging AI and machine learning (ML) to derive insights from data, these technologies are not the only solutions, and it’s important to remember results can be biased or skewed. While AI and ML offer tremendous potential for solving complex problems and making driven decisions, they come with inherent limitations and challenges that require consideration.
Quality outputs come from quality inputs.
First and foremost, AI and ML algorithms are adept at processing large volumes of data and identifying patterns. For the primary language learning models, the datasets are vast, covering multiple points of view. There can be biases in the source data, but the preponderance of data trends toward a balanced view. For private AI, however, its effectiveness is directly proportional to the quality of training data. This is where human intervention becomes crucial, as AI cannot replicate the depth of human experience and judgment.
While AI, especially chat-based AI and LLMs, excel at processing vast datasets, it doesn’t always have the capacity for context-sensitive analysis and the nuanced understanding of domain-specific knowledge that human experts bring. Poor-quality or biased data can lead to skewed results and inaccurate conclusions, compromising the reliability of AI-driven insights. For example, decisions based on skewed data can result in unreliable products that do not meet customer and market needs, which can diminish trust and confidence in the business. Poor quality data can also cause operational delays and supply chain disruptions, wasting valuable time, effort and resources. This underscores the significance of data quality management, human intervention and validation processes in ensuring the integrity of the data used for training and inference.
Read the rest of the article on Forbes.com.
Connect with Media Relations
Please email Walt & Co. for interview or information requests.